Monitoring the training process in team sports
From description to prescription: Part 7
The American Nobel Prize winner Herbert Simon formulated three major goals for scientists:
- Basic science: Knowing what happened (descriptive analysis) and understanding why this happened (diagnostic analysis)
- Applied science: Inferring or predicting what will happen (predictive analysis)
- Science as art: Converting complex data into simple and meaningful information (prescriptive analysis)
These objectives can be applied to the modern sport scientist or practitioner. In our previous blog posts, several topics were discussed relating to ‘basic science’. We used the training process model of Impellizzeri et al. (2019) as a framework.
First, we discussed the different types of indicators that can be used to describe what happens: 1) during the training (i.e. external and internal load) and 2) as a result of the training (i.e. training outcomes).
Next, to better understand why things happen, we aimed to provide insights into the relationships between these indicators. The relation between external and internal load and the relation between load and training outcomes such as performance, fitness, fatigue, and injury risk were discussed.
Sport scientists and practitioners often examine these relationships by using data that is collected in the past. However, to increase the impact on the training process, their primary goal is to use their knowledge and understanding in making inferences or predictions about what will happen in the future (‘applied science’).
By reducing the complexity of these inferences and predictions, the sport scientist and practitioner subsequently aims to communicate their practical advice to other staff members in the most efficient and effective way (science as art). We will discuss ‘science as art’ in our next posts. In the current blog we focus on the goal of ‘applied science’ and in particular on predictive analysis.
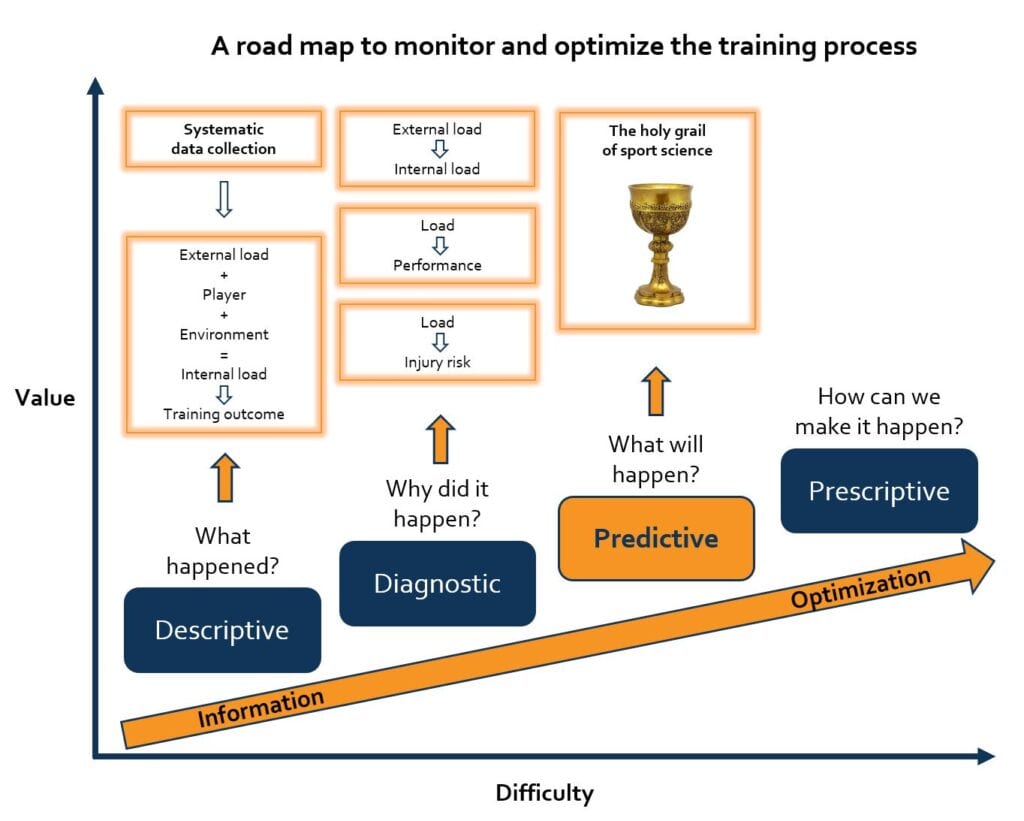
Let’s first explain the difference between inference and prediction. Inferential analysis is considered as modelling possible causal relationships between input (e.g. training load) and output variables (e.g. injury risk) and using this forthcoming information to estimate what will happen in the future.
In practice, one may find a causal relationship between high-speed running and hamstring injury risk, which can be used to manage high-speed running during training. Such relationships are, however, often mediated and/or moderated by other variables. Mediating variables are intermediary steps that explain the relationship between the input and output variable (e.g. high-speed running → muscle stretch → hamstring injury risk). Moderating variables modify the relationship between input and output variables (e.g. previous hamstring injury) (link to article).
Given the complex interplay between inputs, mediators, moderators and resulting outputs, such models often include a lot of interrelated variables. This makes it challenging to accurately manage these variables to achieve positive outcomes.
In predictive analysis, one is not per se interested in the causal explanation of output variables, but more on the model’s ability to accurately predict future outputs based on a set of input variables, with predictive accuracy improving with an increasing number of input variables (figure 2b). This does, however, often result in a black box approach in which it is not clear how the different inputs cause the output.
To further illustrate the difference between inference and prediction, we will now discuss both elements with regard to two of the most known and easy-to-apply training load models in sports science.
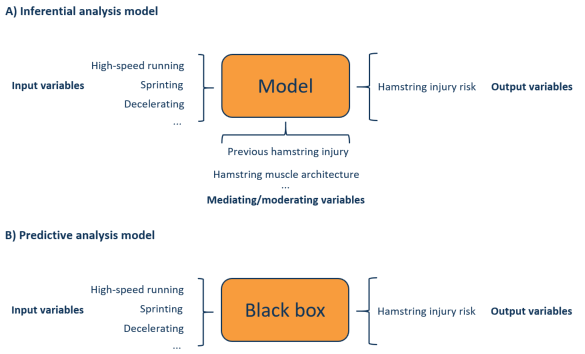
Adapted from Jovanovic, 2017
The impulse-response model of Banister, explained in our third blog, describes the relationship between load and performance. Load is considered as the input variable, performance is considered as the output variable, and fitness and fatigue are considered as the positive and negative mediating variables.
The relationship between these variables are described by two mathematical factors, which reflect the magnitude of fitness and fatigue effects from the load (k) and the decay of these effects over time (Ƭ). This model was developed based on inferential analysis, showing that fitness evolves slowly and fatigue evolves more rapidly. Therefore, one can infer that to optimise performance, the fatigue effects need to be minimised while the fitness effects need to be maximised.
During the preparation towards an important game, one could therefore decrease the training load in order to achieve a rapid decrease in fatigue and a relatively small decrease in fitness, resulting in an improved performance (i.e. tapering strategy).
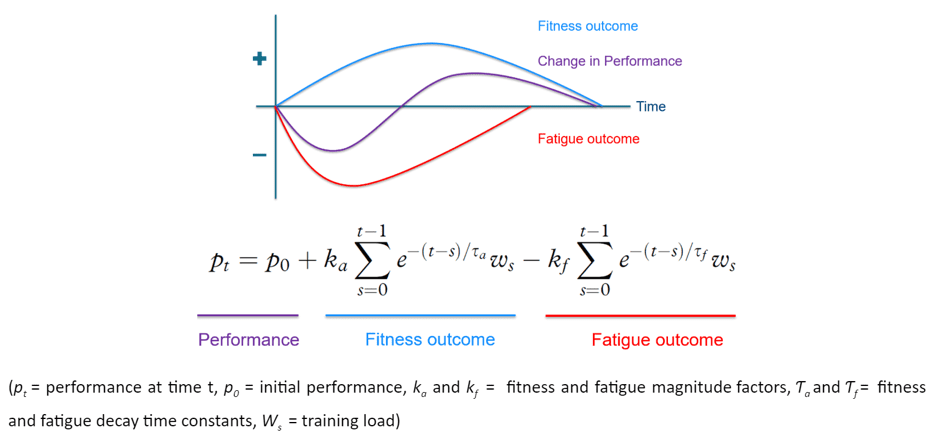
As explained in our previous blog post, this model received some criticism by not including multiple input variables or taking into account moderating variables. Such extra information is important to manage the k and Ƭ factor for each individual player and training context. To obtain the best fit between the modelled and the actual performance, a retrospective analysis can be performed to find the most suitable k and Ƭ factors. However, a high variability in these factors between players is observed. The use of general models is therefore not advised. Although the fit between the individually modelled and the actual performance may be appropriate (which is not always the case), this does not mean that the model is able to accurately predict future performance. This indicates that the most suitable k and Ƭ factor fluctuate over time. The impulse-response model of Banister can be considered a useful tool to model performance by inferring the effects of load on fitness and fatigue, and subsequent performance. However, the model is not able to accurately predict future performance, indicating that it must be interpreted with caution.
In our second example, we focus on the acute-chronic workload ratio (ACWR), discussed in our sixth blog (link to article). This model describes the relation between load and injury risk (link to article). The ACWR is calculated as the ratio between the acute load (e.g. average load last 7 days) and the chronic load (e.g. average load last 28 days). When the acute load is relatively higher than the chronic load, this is interpreted as “the player is doing more than he is normally used to”. Therefore, one can infer that injury risk is increased when the discrepancy between acute and chronic load is high. The ACWR was originally proposed as a model that accurately predicts injury risk. This assumption, however, received much criticism. Two studies examined the predictive ability of the ACWR using the rating of perceived exertion (RPE) as the load input variable. The study of McCall et al. (2018) (link to article) found some association between the ACWR and injury risk, upon which may infer that ACWR is a useful tool for injury prevention (which is currently heavily discussed, for more information, we refer to the following article (link to article)). However, this study also demonstrated that the predictive ability of the ACWR is poor, which means that the ACWR does not accurately classify players as “going to be injured”. A similar finding was found in the study of Fanchini et al. (2018) (link to article).
These two examples highlight the difficulty of predicting training outcomes such as performance and injuries by means of isolated load models. Although these models are often used to plan future training, it is important to understand that one could only infer what will happen but not predict what will happen. The reason for this failed predictive ability can be found in the distinction between these isolated load models and the complex nature of the human body and its environment. The interplay between input, mediating, moderating and output variables is considered as a complex web of determinants, interconnected by non-linear interactions/relationships. Isolated load models fail to recognise these relationships. When the number of explaining variables and its relationships increases, this information may also exceed the capacity of the human brain to understand these relationships. Artificial intelligence could play a role in exploring predictive load models. However, as explained previously, these models often have the disadvantage to be a ‘black box’.
Accurate injury prediction is often used as a sales pitch. Although we have faith in the future development of predictive models, we question the current ability to accurately predict injuries (or other training outcomes). We therefore advise to be cautious when applying predictive models. To illustrate, a high rate of false positives (prediction = injured, but actual observation = not injured) is often observed (referentie zoeken). When practitioners would follow these predictions by modifying the training plan to prevent the predicted injury, this could result in a high number of ineffective and unnecessary interventions (e.g. less (tactical/technical) training exposure). Thus, awaiting future advancements, it remains important to cautiously interpret the different types of data based on the context, current scientific evidence and the practitioners’ own practical experience. Therefore, we prefer to speak about data-informed decision-making instead of data-driven decision-making.
Interested in load monitoring?
Want to see how our platform can help you easily monitor training load with RPE?
Care to keep updated?
Subscribe to our newsletter.
You'll get updates about our recent blog posts and platform updates.